Systematic review on AI for ADAS tasks on platforms with limited resources
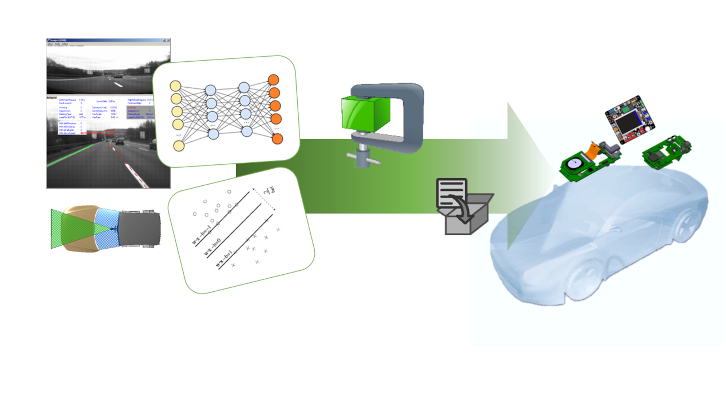
The automotive sector continues moving towards the autonomous car, proposing new improvements at technological level. A significant part of the advances comes from incorporating Artificial Intelligence (AI) through the efficient implementation of neural networks in specific application chips, which allows the vehicle to implement different essential tasks, such as the detection of traffic lights and signals, road margins, pedestrians, cyclists, motorcycles, cars, buses, trucks and other vehicles. The Department of Microelectronics and Electronic Systems at the UAB has carried out a systematic review of the existing solutions to evaluate the advancement of this technology. Their conclusions point to the need to increase research in this area in order to make the implementation of AI algorithms in automobile electronics more efficient in terms of precision, dimensions and energy consumed.
Artificial intelligence and one of its branches, machine learning, has provided fundamental advances in a wide variety of application fields: from medicine to automotive, through the entertainment industry and home automation. Tasks such as the detection and segmentation of elements in the image, the recognition of gestures and activities, as well as the translation and processing of natural language are examples of the tasks in which this type of algorithm has proven its usefulness and performance.
However, not in all cases the application of machine learning algorithms is straightforward and free of technical problems. The computation and memory requirements vary from algorithm to algorithm, making it impossible in some cases to directly implement hardware with limited resources (memory, computation, cost). This is the case of neural networks, a type of machine learning model, which initially required resources not achievable by a microcontroller such as those used in the automotive industry. It should be noted that the limitation in resources is due to the fact that the device, being arranged in and for a car, has more requirements than in other environments: size, durability, resistance to temperature and price, among others.
For this reason, there have been sectors in which the application has been more complex. This is the case of ADAS systems (Advanced Driver Assistance System), in which all the applications that are responsible for improving safety and facilitating the driving task are embedded, in what has been called the autonomous car. Examples of activities are the detection of traffic lights and signals, the detection of the road margins, brake assistance and car-driver interaction, among many others.
Due to this problem, in the last decade methods and platforms have been developed to be able to transfer machine learning algorithms to platforms with limited resources. Thanks to a collaboration between the Kostal Eléctrica company and the TECNIO CEPHIS Center, from the Department of Microelectronics and Electronic Systems of the UAB, a study has been carried out on what is the state of the art regarding the problem of carrying learning models automatic to the hardware (limited resources) used in the ADAS environment and different from PCs and mobiles.
Among the conclusions obtained, two points stand out. The first is the confirmation of the incipient development of specialized libraries for the implementation of neural networks in hardware with limited resources, as well as their release as open source (CMSIS-NN, Tensorflow for Microcontrollers, Embedded Learning Library, uTensor, among others). The second is the deficit of academic, coherent and structured research that allows the validation and comparison of methods, technologies and results, a fact that could indicate the confidential or proprietary nature of many of these developments.
For this reason, it is necessary that there be a greater number of comparable investigations, both at the software, hardware and data level, that allow a coherent and public advance on this subject that will mark the near future of the automotive sector.
Department of Microelectronics and Electronic Systems.
Universitat Autònoma de Barcelona.
References
Borrego-Carazo, Juan; Castells-Rufas, David; Biempica, Ernesto; Carrabina, Jordi. Resource-Constrained Machine Learning for ADAS: a Systematic Review. (2020) 1.1 IEEE Access. DOI: 10.1109/ACCESS.2020.2976513